Artificial Intelligence (AI) is revolutionizing various industries, making high-quality AI training datasets essential for effective model training. Understanding the significance of AI datasets, alongside effective AI dataset strategies, is crucial for harnessing AI’s full potential and driving future technology innovations.
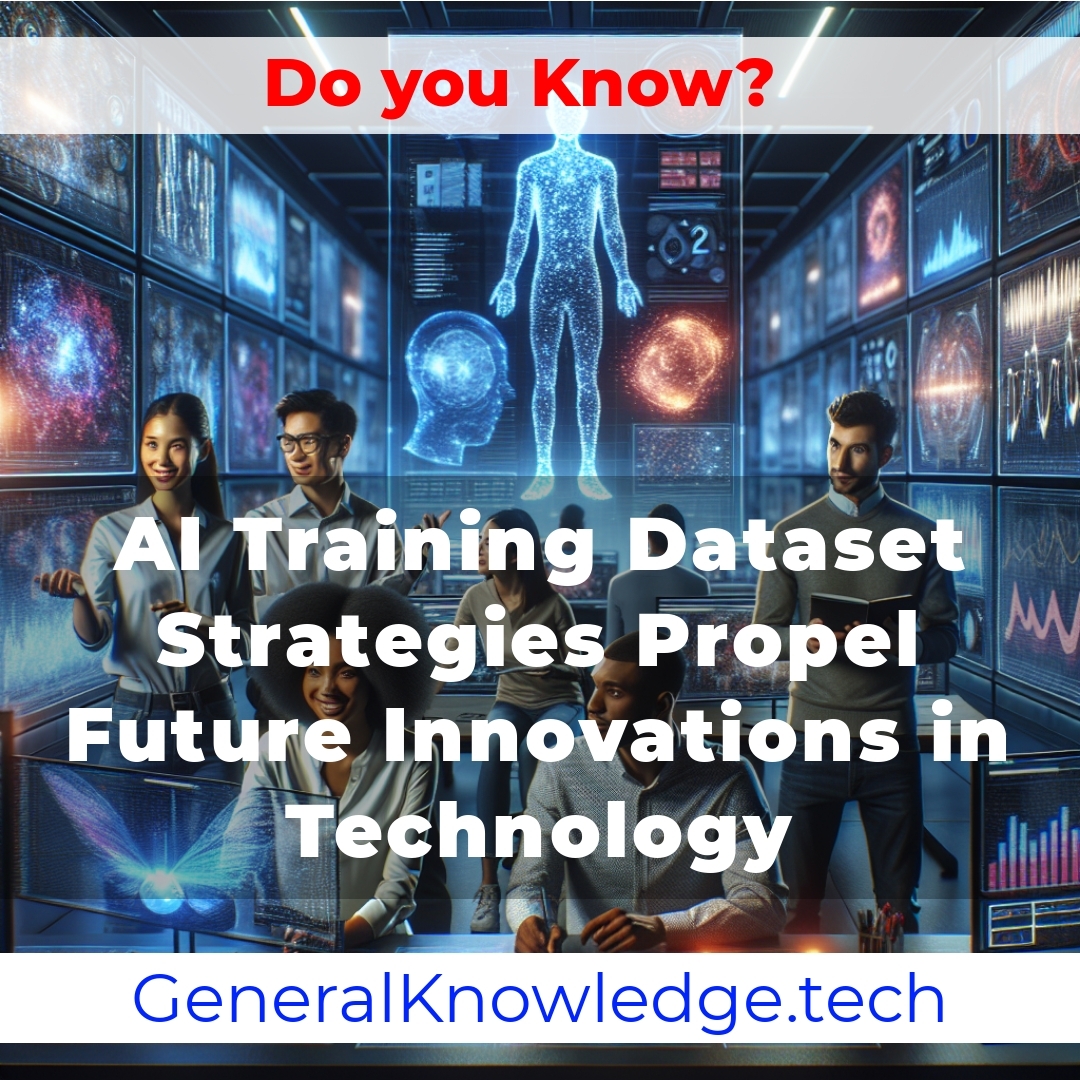
## What Are AI Training Datasets?
AI training datasets are collections of data specifically designed for training machine learning models. These datasets can include diverse types of data such as images, text, and audio, tailored to meet the objectives of various AI applications. The process of compiling these AI training datasets involves gathering data from multiple sources to create a robust and comprehensive training set.
Different types of machine learning datasets play crucial roles in AI training, such as supervised, unsupervised, and semi-supervised datasets. Supervised datasets contain labeled input-output pairs, making it easier for algorithms to learn, while unsupervised datasets consist of unlabeled data that allows models to discover patterns independently. Specialized datasets are also created for specific tasks, like natural language processing or computer vision, each contributing to the broader landscape of artificial intelligence advancements.
## The Importance of Data Quality in AI
In the realm of AI training datasets, data quality is paramount. The effectiveness of an AI model largely hinges on the quality of the training data it receives. Factors such as accuracy, completeness, and relevance are critical when evaluating a dataset.
High-quality, clean, and well-annotated datasets are vital for successful AI training. Datasets filled with errors or irrelevant information can lead to subpar model performance, resulting in inaccurate predictions and misguided decisions. Therefore, ensuring superior data quality is essential for developing reliable AI models that contribute to future technology innovations.
## Best Practices for Creating Effective AI Training Datasets
Leveraging effective AI dataset strategies is key to creating high-quality AI training datasets. Here are some best practices to follow:
– **Gather Diverse Data**: Curate datasets that encompass a wide range of scenarios. This diversity helps models generalize better to unseen data, ultimately enhancing their performance.
– **Label Data Accurately**: Proper labeling is crucial for the success of AI training datasets. Utilize clear guidelines and involve multiple annotators to ensure consistency and accuracy in annotations.
– **Regularly Update Datasets**: The world is dynamic; hence, your AI training datasets should reflect current realities. Regular updates keep the model relevant and effective in real-world applications.
– **Set Aside Test Data**: Always reserve a portion of your dataset for testing. This practice allows for an objective evaluation of the model’s performance after training.
By adhering to these best practices for AI training datasets, organizations can significantly improve model accuracy and reliability.
## Strategies for Collecting Quality AI Training Data
Employing effective strategies for collecting AI training data ensures the creation of high-quality datasets that enhance model performance. Consider these techniques:
– **Crowdsourcing**: Engaging the public for data collection can yield vast amounts of high-quality data from diverse sources, enriching your AI training datasets.
– **Web Scraping**: Extracting data from websites can provide valuable information for your AI datasets, provided that you adhere to legal and ethical guidelines.
– **Synthetic Data Generation**: Creating artificial datasets through simulations or algorithms can help fill gaps in real data, particularly in scenarios where data scarcity is a challenge.
– **Efficient Data Annotation**: Leverage tools and platforms that streamline the annotation process, improving both efficiency and accuracy in your AI training datasets.
Implementing these AI dataset strategies will lead to a well-rounded and effective dataset that enhances model performance and supports future technology innovations.
## Real-World Examples of Successful AI Training Dataset Implementation
Examining real-world case studies demonstrates the effectiveness of robust AI training dataset strategies:
1. **Healthcare Sector**: A leading healthcare company developed an AI model for early disease detection by implementing a comprehensive AI training data collection strategy. This included gathering diverse patient data, ensuring accurate annotations from healthcare professionals, and regularly updating their dataset. The outcome was a highly accurate AI model that significantly improved patient outcomes.
2. **Automotive Industry**: An automotive company successfully trained its self-driving algorithms using synthetic data. By generating a variety of driving scenarios, they thoroughly tested their systems, ensuring safety in real-world applications.
These examples underscore the critical role that high-quality AI training datasets play in driving artificial intelligence advancements and highlight effective strategies for data collection.
## Conclusion
In conclusion, the importance of high-quality AI training datasets cannot be overstated. Well-defined datasets, along with a focus on data quality, best practices, and strategic collection methods, are crucial for successful AI model training. As artificial intelligence continues to evolve, the significance of these elements will ensure that models remain accurate, reliable, and relevant in an ever-changing technological landscape. Emphasizing the role of quality datasets in AI training is vital for maximizing the potential of artificial intelligence across various industries and propelling future technology innovations.
### FAQs
**What is an AI training dataset?**
An AI training dataset is a collection of data used to train machine learning models, including various types of data such as images, text, or audio, based on the objectives of the AI application.
**What types of datasets are used in AI training?**
AI training datasets can be categorized into several types:
– **Supervised datasets**: Contain labeled input-output pairs.
– **Unsupervised datasets**: Comprised of unlabeled data for models to discover patterns.
– **Semi-supervised datasets**: Combine elements of both supervised and unsupervised datasets.
**Why is data quality important for AI training?**
Data quality is critical as it directly impacts the performance of AI models. Ensuring factors like accuracy, completeness, and relevance is vital to avoid poor predictions and flawed decisions.
**What are some best practices for creating AI training datasets?**
– Gather diverse data to enhance model generalization.
– Label data accurately and consistently.
– Regularly update datasets to maintain relevance.
– Set aside a portion for testing performance.
**What strategies can be used to collect AI training data?**
Effective strategies include:
– **Crowdsourcing**: Engaging the crowd for diverse data.
– **Web scraping**: Extracting data from websites.
– **Synthetic data generation**: Creating artificial datasets.
– **Data annotation**: Utilizing tools for efficient annotation.
**Can you provide examples of successful AI training datasets?**
Sure! Examples include:
– A healthcare company that improved patient outcomes through diverse data and accurate annotations for disease detection.
– An automotive company that ensured safety in self-driving algorithms by training with synthetic data.
By implementing these AI training dataset strategies, organizations can propel future innovations in technology and maximize the potential of artificial intelligence.