Machine learning in healthcare is at the forefront of a transformative wave that is reshaping the medical landscape. By integrating advanced machine learning innovations into various healthcare domains, we can significantly enhance diagnostic accuracy, predict patient outcomes, and streamline patient management. This article delves into the pivotal role of machine learning in healthcare innovation, particularly as it is being pushed forward by MIT’s groundbreaking research.
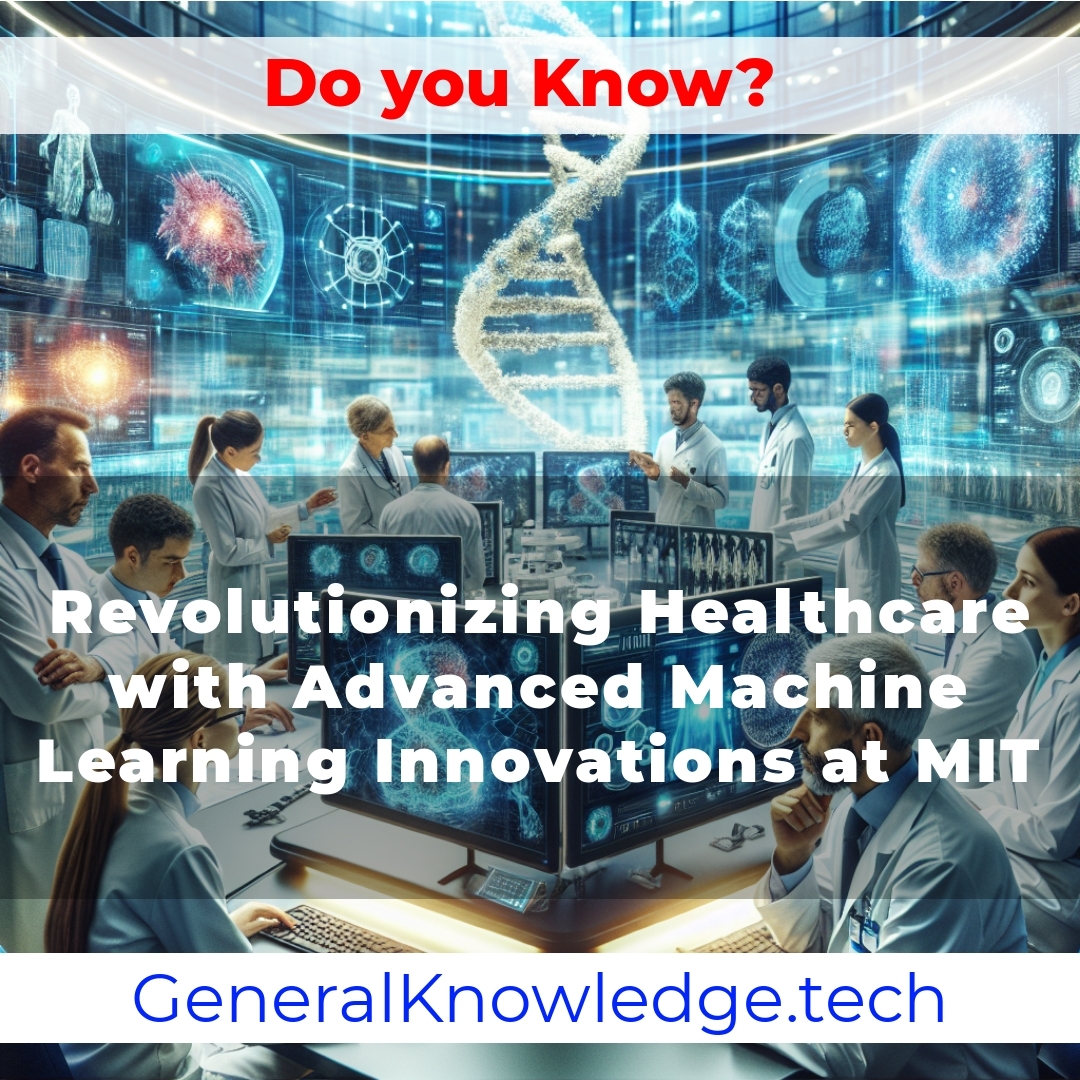
## The Evolution of Healthcare Technology
Technology has consistently played a crucial role in healthcare innovation. From the invention of the stethoscope to the implementation of digital health records, the evolution has been remarkable. Recently, the introduction of advanced machine learning systems has fundamentally transformed our approach to medical challenges. This class of technology empowers us to analyze vast amounts of health data, leading to improved patient outcomes and streamlined processes.
Machine learning in healthcare not only enhances diagnostics but also drives predictions for patient management. These advancements enable healthcare providers to make informed decisions, ultimately leading to notable improvements in health systems worldwide.
## How Machine Learning is Transforming Healthcare
When discussing how machine learning is revolutionizing healthcare, several key areas emerge as particularly impactful:
### Diagnostics
Advanced machine learning plays a vital role in healthcare diagnostics. By utilizing powerful algorithms to analyze medical images, we can achieve unprecedented accuracy in identifying conditions. This reduction in misdiagnoses paves the way for more effective treatments and better patient outcomes.
### Treatment Prediction
In the realm of treatment prediction, machine learning systems evaluate patient data to forecast the most effective treatment options. For instance, predictive analytics in healthcare allows doctors to gain insights into potential outcomes for treatments specifically tailored to individual patients, driving healthcare innovation forward.
### Patient Management
On the patient management front, machine learning optimizes workflows and enhances communication within healthcare facilities. Automated systems ensure that patient records are updated in real-time, allowing healthcare providers to concentrate on delivering quality care.
## Case Studies on Machine Learning in Medical Technology
Real-world examples highlight the power of machine learning in healthcare innovation.
### Case Study 1: Enhancing Diagnostic Accuracy
In a notable case, an AI system analyzed thousands of dermatology images, diagnosing skin cancer with an accuracy rate comparable to that of experienced dermatologists. This success underscores the necessity of integrating advanced machine learning technologies into standard medical practices.
### Case Study 2: Predicting Patient Outcomes
Another compelling study showcased artificial intelligence in medicine to predict hospital readmission rates. By analyzing a range of patient data, the system identified individuals at risk for readmission, enabling healthcare providers to implement preventive measures, leading to significant health improvement outcomes.
## The Role of Digital Health Solutions
Digital health solutions powered by machine learning are increasingly prevalent, offering benefits for both healthcare providers and patients. For example, telemedicine platforms often utilize advanced machine learning algorithms to personalize treatment plans based on individual data, making healthcare more accessible and efficient.
Looking ahead, we can anticipate greater integration of healthcare technology into our daily lives. With advancements in digital health solutions, a more proactive approach to health management will emerge, improving overall health outcomes.
## Challenges and Considerations
While the integration of machine learning in healthcare presents numerous benefits, it also introduces challenges:
– **Adoption Barriers:** Many healthcare facilities face obstacles in implementing advanced systems due to high costs and necessary training.
– **Ethical Issues:** Alongside the potential benefits, ethical considerations arise, particularly regarding data privacy. Protecting patient data is paramount.
– **Training and Validation:** Ensuring machine learning systems are well-trained and validated is crucial. Errors in these systems can have severe consequences in healthcare environments.
## Conclusion
The impact of machine learning in healthcare is profound. As explored throughout this article, advanced machine learning technologies are transforming our approach to healthcare by improving diagnostics, predicting patient outcomes, and enhancing patient management. As technology continues to evolve, the potential for positive change in healthcare appears limitless.
This is an exciting time for healthcare innovation, driven by machine learning systems. The future of healthcare promises incredible advancements, creating a healthier world for everyone.
## Call to Action
We want to hear from you! Have you encountered experiences related to machine learning in healthcare? Share your thoughts or questions in the comments below. For those interested in exploring the intersection of technology and healthcare further, check out various online resources, courses, and webinars. Let’s engage in discussions about the exciting advancements in healthcare technology!
### FAQ
**What is the role of machine learning in healthcare?**
Machine learning enhances various aspects of healthcare, including diagnostics, treatment prediction, and patient management. It enables healthcare providers to analyze large datasets, leading to improved patient outcomes and more efficient processes.
**How does machine learning improve diagnostics?**
Machine learning algorithms can analyze medical images with high accuracy, helping identify medical conditions and reducing misdiagnoses, resulting in more effective treatments.
**What is treatment prediction and how does it work?**
Treatment prediction uses machine learning to evaluate patient data and forecast the most effective treatment options, allowing doctors to choose personalized treatments based on potential outcomes.
**Can you provide examples of machine learning in medical technology?**
– **Case Study 1:** An AI system analyzed dermatology images and diagnosed skin cancer with accuracy similar to experienced dermatologists.
– **Case Study 2:** A machine learning system predicted hospital readmission rates, allowing for preventive measures that improved health outcomes.
**What are digital health solutions and how do they benefit healthcare?**
Digital health solutions powered by machine learning, like telemedicine platforms, personalize treatment plans based on individual data, enhancing healthcare accessibility and efficiency.
**What challenges come with integrating machine learning into healthcare?**
– **Adoption Barriers:** High costs and training requirements can hinder implementation in healthcare facilities.
– **Ethical Issues:** Data privacy concerns necessitate compliant handling of patient data.
– **Training and Validation:** Proper training and validation of machine learning systems are crucial to avoid errors that could negatively impact patient care.